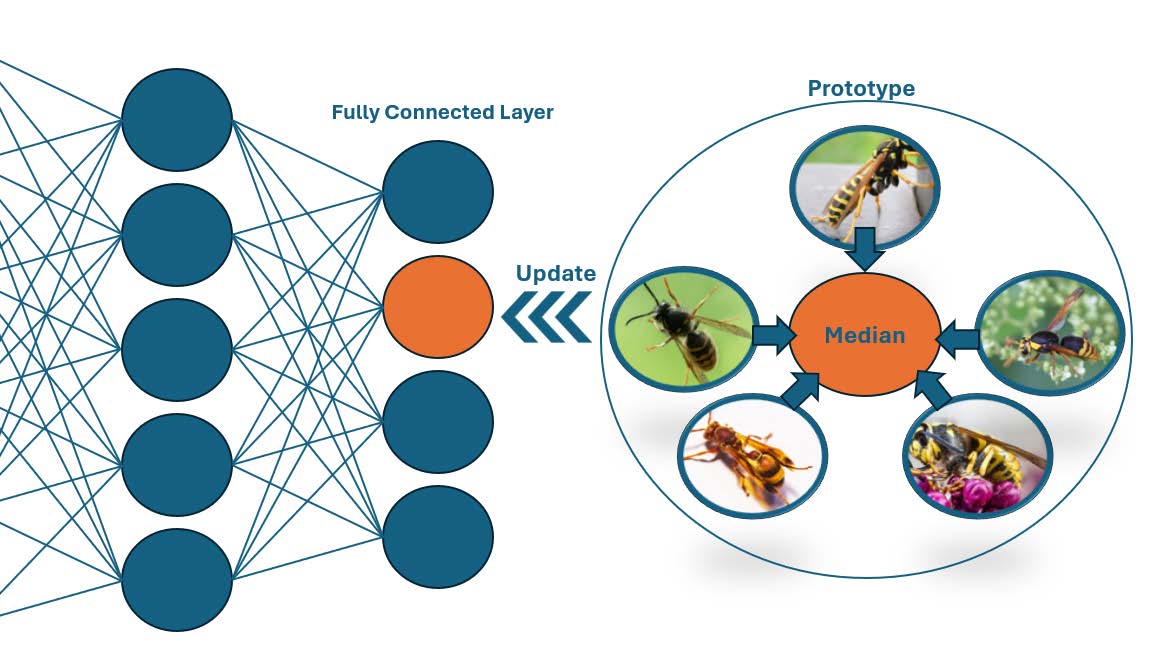
Agricultural productivity is heavily impacted by pests such as aphids, which cause significant crop damage and act as vectors for plant diseases. Effective pest detection and management are crucial but challenging with traditional methods. This research introduces the Attention and Median Training-Free Prototype Calibration (AM-TEEN) model, a novel Few-Shot Class
Incremental Learning (FSCIL) approach designed to improve the accuracy and robustness of pest detection systems. The model was evaluated using datasets including Aphids, Agricultural Pests from Kaggle, DLFautoinsects, and CIFAR-100. The experimental setup involved systematically varying one parameter at a time to comprehensively analyze its impact on model performance. AM-TEEN model significantly outperforms the base TEEN model. Specifically, in the first incremental session, accuracy improved from 87.50% to 97.92%, and in the last incremental session, accuracy increased from 67.08% to 74.17%. These improvements underscore the model’s enhanced ability to handle incremental learning challenges and maintain high accuracy across diverse datasets. The enhanced model not only offers better accuracy and robustness but also demonstrates the potential for more efficient and scalable pest detection systems.
Agricultural productivity is heavily impacted by pests such as aphids, which cause significant crop damage and act as vectors for plant diseases. Effective pest detection and management are crucial but challenging with traditional methods. This research introduces the Attention and Median Training-Free Prototype Calibration (AM-TEEN) model, a novel Few-Shot Class
Incremental Learning (FSCIL) approach designed to improve the accuracy and robustness of pest detection systems. The model was evaluated using datasets including Aphids, Agricultural Pests from Kaggle, DLFautoinsects, and CIFAR-100. The experimental setup involved systematically varying one parameter at a time to comprehensively analyze its impact on model performance. AM-TEEN model significantly outperforms the base TEEN model. Specifically, in the first incremental session, accuracy improved from 87.50% to 97.92%, and in the last incremental session, accuracy increased from 67.08% to 74.17%. These improvements underscore the model’s enhanced ability to handle incremental learning challenges and maintain high accuracy across diverse datasets. The enhanced model not only offers better accuracy and robustness but also demonstrates the potential for more efficient and scalable pest detection systems.
Link to the paper of this project.